Open-Source LLMs vs. Proprietary Giants: Democratizing AI and Closing the Gap
- sujosutech
- Mar 20
- 4 min read
Introduction: The Rise of Open-Source LLMs
The artificial intelligence (AI) landscape has long been dominated by proprietary large language models (LLMs) from tech giants like OpenAI, Google, and Anthropic. However, the emergence of open-source LLMs is reshaping the field, making advanced AI more accessible, customizable, and transparent. Open-source models are breaking down barriers, enabling researchers, startups, and developers to harness cutting-edge AI without the restrictions imposed by closed models. In this article, we’ll explore how open-source LLMs are democratizing AI, compare them with proprietary models, and provide a detailed comparison of the top open-source LLMs available today, including the latest LLaMA 3.3.
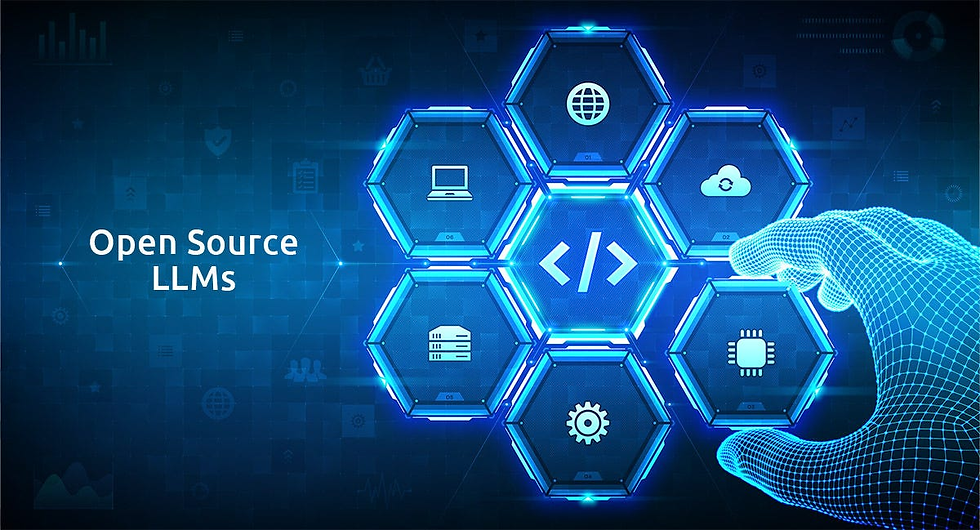
1. Open-Source LLMs: Democratizing AI
Open-source LLMs are transforming the AI ecosystem by fostering innovation, collaboration, and inclusivity. Unlike proprietary models, which operate behind closed doors, open-source LLMs allow anyone to inspect, modify, and fine-tune them for specific use cases. Here’s how they contribute to AI democratization:
Key Benefits of Open-Source LLMs:
Accessibility: Open-source models eliminate the high costs associated with API-based proprietary models, making advanced AI accessible to startups, researchers, and hobbyists.
Free access to state-of-the-art models enables experimentation and innovation without financial barriers.
Customization: Developers can fine-tune these models for specific applications, optimizing them for tasks like code generation, multilingual support, or reasoning.
Open-source models allow for modifications to the architecture, training data, and inference pipelines, enabling tailored solutions.
Transparency & Security: Open-source projects allow researchers to audit the models for biases, vulnerabilities, and ethical concerns, fostering trust and accountability.
Transparency in model design and training data helps address issues like bias, misinformation, and misuse.
Community-Driven Innovation: A global community contributes to improving these models, leading to faster advancements and a diverse range of applications.
Platforms like Hugging Face, EleutherAI, and Meta’s LLaMA initiative have created ecosystems where developers can share models, datasets, and tools.
Examples of Democratization in Action:
Education: Open-source LLMs are being used to teach AI and NLP concepts, with projects like Hugging Face’s educational resources and Google’s Gemma.
Research: Researchers are leveraging open-source models to conduct experiments and publish findings without relying on proprietary systems.
Startups: Small businesses and startups are using open-source LLMs to build AI-powered applications, reducing dependency on expensive proprietary APIs.
2. Open-Source LLMs vs. Proprietary Models
While proprietary models like GPT-4, Claude 3, and Google Bard offer superior performance out-of-the-box, open-source LLMs provide unmatched flexibility and customization. Here’s a detailed comparison of key aspects:
Comparison Table
Parameter | Open-Source LLMs | Proprietary LLMs |
Model Size | Typically smaller (e.g., LLaMA 2: 7B-70B parameters). | Larger and more optimized (e.g., GPT-4: rumored to have >1T parameters). |
Training Data | Often trained on publicly available datasets, which may be limited in scope. | Trained on vast, diverse, and proprietary datasets, enabling better generalization. |
Inference Speed | Depends on hardware; slower on consumer-grade GPUs. | Optimized for fast inference via scalable cloud infrastructure. |
Accuracy and Coherence | Good for specific tasks but may struggle with complex reasoning and long contexts. | Superior in handling complex queries, maintaining context, and generating coherent responses. |
Fine-Tuning Capabilities | Full control over fine-tuning; supports domain-specific adaptation. | Limited to API-based fine-tuning or prompt engineering. |
Multimodal Capabilities | Limited; most open-source models are text-only. | Proprietary models (e.g., GPT-4 Vision) support text, images, and other modalities. |
Bias and Fairness | Users must address biases manually; no built-in safeguards. | Providers implement alignment techniques to reduce bias and harmful outputs. |
Scalability | Requires significant infrastructure for scaling. | Easily scalable via provider’s cloud infrastructure. |
Robustness | May produce inconsistent or incorrect outputs without extensive fine-tuning. | Highly robust, with fewer hallucinations and errors |
Energy Efficiency | Less optimized; higher energy consumption per query. | Highly optimized for energy efficiency in large-scale deployments. |
Startups and Researchers: Open-source models are ideal for cost savings and customization.
Enterprises: Proprietary models may be preferred for their reliability, performance, and ease of use.
3. Top Open-Source LLMs Available Today
Several open-source LLMs have emerged as competitive alternatives to proprietary models. Below are some of the most notable ones:
LLaMA 3 & LLaMA 3.3 70B (Meta) – The latest iteration from Meta, optimized for efficiency and improved reasoning.
Mistral 7B & Mixtral 8x7B (Mistral AI) – Highly efficient models with strong performance in reasoning tasks.
Falcon 180B (Technology Innovation Institute) – A massive model with excellent comprehension and fluency.
Gemma 2 & Gemma 3 Vision (Google DeepMind) – Vision-enabled models optimized for multimodal applications.
DeepSeek R1 (DeepSeek AI) – A well-balanced model with robust mathematical reasoning capabilities.
4. Challenges and Future of Open-Source LLMs
Despite their advantages, open-source LLMs face challenges like high computational requirements and ethical concerns. However, the future looks promising, with trends like:
Smaller, More Efficient Models: Models like Gemma and Mistral are leading the way in efficiency.
Improved Multilingual and Multimodal Capabilities: LLaMA 3.3 and BLOOM are pushing the boundaries in multilingual support.
Increased Adoption in Industry: Open-source LLMs are being integrated into commercial applications at an unprecedented rate.
5. How Sujosu is Leveraging Open-Source LLMs
At Sujosu Technology, we are actively utilizing state-of-the-art open-source LLMs to enhance various applications, including:
Document Intelligence – Fine-tuning models like LLaMA 3 and Mixtral 8x7B to improve document classification, summarization, and extraction.
Audio Transcription – Leveraging models such as Whisper and DeepSeek R1 to enhance speech-to-text capabilities.
Chatbots & Virtual Assistants – Implementing open-source chat models to create intelligent, cost-effective virtual assistants.
Custom AI Solutions – Helping clients fine-tune and deploy open-source models for their specific needs, reducing costs and improving efficiency.
Beyond our current work, open-source LLMs can be applied to numerous domains, including:
Healthcare – Medical transcription and AI-assisted diagnosis using fine-tuned language models.
Legal Industry – Automated contract review and legal document analysis.
Finance – AI-driven market analysis and fraud detection.
Education – Personalized learning assistants and tutoring systems.
E-commerce – AI-powered recommendation systems and customer support automation.
Our team at Sujosu Technology is well-equipped to guide businesses in integrating and optimizing open-source LLMs for their unique requirements. By leveraging these models, we help organizations achieve cost savings while maintaining high-performance AI solutions.
Conclusion
Open-source LLMs like LLaMA 3.3, Mistral, Gemma, and DeepSeek are democratizing AI and challenging proprietary models. While proprietary models still lead in performance, open-source models offer unmatched flexibility, transparency, and cost-effectiveness. Whether you’re a researcher, developer, or business, there’s an open-source LLM tailored to your needs. Explore these models today and join the movement to democratize AI!.
Comentarios