Bridging the Gap: How the Model Context Protocol (MCP) Powers Smarter AI Agents
- sujosutech
- Apr 14
- 4 min read
Updated: Apr 15
Today’s enterprises are no longer just exploring AI—they’re integrating it deeply into workflows. But for AI to truly drive business outcomes, it must do more than answer queries. It should understand context, access real-time data, and interact with tools just like your team does.
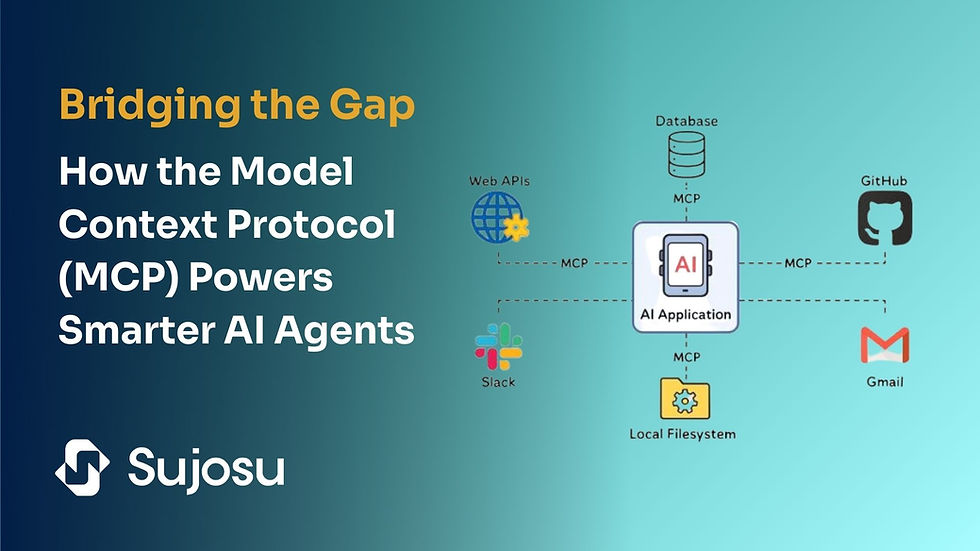
This is where the Model Context Protocol (MCP) plays a pivotal role.
At Sujosu Technology, we help organizations build intelligent systems by integrating protocols like MCP that allow Large Language Models (LLMs) to move beyond static reasoning and actively engage with your tools, data, and systems in real-time.
Why Traditional LLMs Fall Short
Even the most powerful LLMs—like GPT-4, Claude, or Gemini—are fundamentally limited in enterprise environments. Without integration, they can’t:
Access live project data or current tool statuses
Understand updated content in files, CRMs, or databases
Interact with APIs, dashboards, or workflow engines
MCP solves this disconnect by creating a secure, standardized way for AI models to fetch data, trigger workflows, and deliver actionable insights—all without breaking context. Think of MCP as the USB-C port for AI — a universal connector that enables AI to securely interface with calendars, databases, APIs, and internal tools, just like plugging into a system with ease and reliability.
How MCP Enhances Real-World AI Workflows
At Sujosu, we’ve worked on MCP-driven use cases across industries. Here are just a few examples of how it improves real-time decision-making:
Developer Support with Real-Time Debugging
Scenario: A developer types, “Why is the payment webhook failing on staging?”
With MCP:
The AI pulls logs from monitoring tools
Cross-checks recent commits
Analyzes feature flags
Returns a clear root-cause summary with suggested resolution
Proactive Sales Assistance
Scenario: A sales rep asks, “Summarize the client’s recent activity before my call.”
With MCP:
The AI retrieves email communication logs
Reviews LinkedIn activity and recent website visits
Analyzes support tickets
Provides a personalized, contextual briefing instantly
Health Assistant with Patient Context
Scenario: A doctor preparing for rounds says, “Summarize this patient’s history before I meet them.”
With MCP:
The AI fetches EHR records, medications, and doctor’s notes
Reviews recent lab results
Summarizes the findings in seconds
Example Response: “John Doe, 62. Type II diabetes, recent rise in blood glucose. Adjusted meds 3 weeks ago. Complained of fatigue. MRI pending. Suggested next step: thyroid function test.”
These examples show how MCP transforms AI into a proactive co-pilot, adapting to roles across development, sales, healthcare, and beyond.
Key Features of Model Context Protocol (MCP)
Standardized Connectivity – One protocol to connect AI models to any application, file system, or API
Real-Time Execution – Enables AI to act on live data, not static training inputs
Built-In Security – Fine-grained permissions, authentication, and access control
Real Implementation Example: Weather Intelligence Powered by MCP
To demonstrate how the Model Context Protocol (MCP) brings real-time intelligence into AI applications, the Sujosu Technology team developed a full-stack weather assistant. This proof of concept showcases how LLMs can fetch and act on live weather data, generate natural language responses, and respond to user queries—all through dynamic tool usage enabled by MCP.
System Architecture:
The application includes three interconnected components, each playing a critical role:
MCP Server – Connects to external weather APIs and exposes tools for the AI to use.
MCP Client – Interfaces with the AI model (e.g., GPT-4o-mini), enabling it to use real-time tools.
Web Server & UI – Serves a user interface and manages API communication.
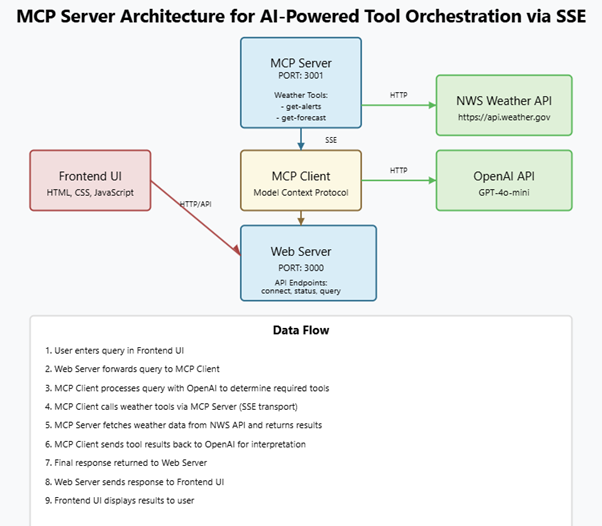
MCP Server
Tools Provided:
get-alerts: Retrieves weather alerts by U.S. state code.
get-forecast: Fetches 7-day forecasts using latitude/longitude.
Communication:
Uses SSEServerTransport with endpoints:
/sse: Streams real-time events.
/messages: Receives incoming tool requests.
External Integration:
Connects with the National Weather Service API to return LLM-friendly, structured JSON data.
MCP Client
Built using @modelcontextprotocol/sdk/client with SSEClientTransport.
Connects to the MCP Server and dynamically registers available tools.
Interacts with the AI model (e.g., GPT-4o-mini) by detecting when to trigger a tool call and embedding live data into the response.
Web Server & API Integration
The frontend is a lightweight static site, with REST API endpoints served from a Node.js backend:
API Endpoints:
POST /api/connect: Initializes the MCP client session and connects to the MCP server
GET /api/status: Returns current MCP client connection status
POST /api/query: Accepts user queries, processes them through the AI model, and returns intelligent, contextual responses
User Experience
The simple web UI allows users to:
Input natural language questions like "What’s the weather in Texas?"
See real-time, streaming responses generated by the AI
Watch how the AI dynamically invokes MCP tools for live data
Example Interaction: User: “Are there any weather alerts in Florida?”
AI Process:
Recognizes the need for real-time alert data.
Calls the get-alerts tool from the MCP server.
Parses the structured response.
Summarizes the alert in natural language — all within seconds.
Security and Reliability Built In
Sujosu’s implementation follows key enterprise-grade best practices:
Strong schema validation using Zod to ensure clean, predictable data
Authentication & role-based access control for tool usage
Robust logging and error recovery mechanisms for high availability
Best Practices for MCP Integration
Schema Validation: Use tools like Zod for consistent data formatting.
Error Handling: Implement fallback logic for tool failures or disconnections.
Monitoring: Log all interactions for traceability and debugging.
Access Control: Enforce fine-grained permissions for tool usage.
Looking Ahead: The Future of MCP in Enterprise AI
As adoption grows, MCP will become central to building advanced AI systems that:
Automate software development tasks
Enable smart customer support
Provide intelligent, real-time decision-making across industries
At Sujosu Technology, we specialize in implementing MCP-driven AI architectures that align with your business goals. Whether you're modernizing legacy systems or building AI-native applications, we’ll help you integrate LLMs with real-time tools, data sources, and workflows securely and efficiently.
Ready to Build AI That Thinks in Context?
Whether you're building a health-tech platform, sales automation tool, or real-time analytics dashboard—Model Context Protocol can transform how your AI interacts with your ecosystem.
Partner with Sujosu Technology to design, develop, and deploy context-aware AI applications that are secure, scalable, and built for the future.
Contact us today to explore how Sujosu can bring your AI systems to life with MCP integration.
Comentarios